Integrating Data Analytics with RPA for Business Innovation: A Case Study
RPA WITH DATA ANALYTICS
Saravanan Pandiaraj and Geetha Sridhar
7/24/20242 min read
Introduction to RPA and Data Analytics
In the contemporary business landscape, leveraging technology is paramount for achieving efficiency and innovation. Robotic Process Automation (RPA) and data analytics are two such technologies that, when combined, can drive significant business transformation. This case study explores the integration of RPA with data analytics, elucidating its potential for fostering business innovation and operational excellence.
Understanding the Synergy Between RPA and Data Analytics
RPA involves the use of software robots to automate repetitive and rule-based tasks, thereby freeing human resources for more strategic activities. On the other hand, data analytics involves examining raw data to draw meaningful insights that inform decision-making. When integrated, RPA can handle large volumes of data swiftly, while data analytics can provide actionable insights from this data, creating a powerful synergy that enhances business processes.
Case Study: Implementation and Outcomes
Consider a financial services company that implemented RPA combined with data analytics to streamline its loan processing workflow. Initially, the company faced challenges with manual data entry, leading to errors and delays. By deploying RPA, the company automated data collection and entry processes. Simultaneously, data analytics tools analyzed this data to identify patterns and predict loan approval likelihood.
The results were remarkable. The automation reduced processing time by 50%, and the predictive analytics improved loan approval accuracy by 30%. This integration not only enhanced operational efficiency but also improved customer satisfaction due to faster and more accurate loan processing.
Challenges and Considerations
While the integration of RPA with data analytics presents numerous benefits, it also comes with challenges. Data quality is a critical consideration, as inaccurate data can lead to erroneous analytics results. Additionally, the initial implementation cost and the need for skilled personnel to manage and maintain these technologies can be significant barriers for some organizations.
To mitigate these challenges, businesses should invest in high-quality data management practices and provide adequate training for their staff. Moreover, a phased implementation approach can help manage costs and allow for gradual adaptation to these technologies.
Conclusion
Integrating RPA with data analytics offers a robust solution for business innovation, driving efficiency, accuracy, and strategic decision-making. This case study highlights the transformative potential of this integration, illustrating how businesses can overcome operational challenges and achieve significant improvements in their processes. As technology continues to evolve, the synergy between RPA and data analytics is set to play an increasingly critical role in shaping the future of business operations.
GreenwaveX Technologies
“From bugs to features, we’ve got you covered.”
Policy
Contact
All rights reserved for GreenwaveX Technologies © 2024.
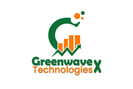

GreenwaveX Technologies
30 N Gould St Ste R Sheridan,Sheridan,
WY 82801.
United States of America.